Google’s advancements in artificial intelligence (AI) have been at the forefront of technological innovation, and Google’s newest AI model, the PaLM 2 large language model, is making waves in the AI community. The PaLM 2 model, as announced by Google, employs nearly five times more text data for training than its predecessor, the LLM (Large Language Model). While Google claims improved efficiency with a smaller model, the lack of transparency surrounding the training data used in AI models is becoming an increasingly debated topic among researchers. In this article, we will delve into the details of Google’s newest A.I. model, exploring the implications of its colossal data usage and the potential it holds for transforming the field of AI.
Google’s newest AI model, PaLM 2: Expanding Training Data:
The PaLM 2 language model represents a significant leap forward in AI capabilities, primarily due to its enhanced training data size. By utilizing nearly five times more text data than its predecessor, Google aims to improve the model’s performance, language understanding, and ability to generate coherent and contextually relevant responses. The abundance of training data allows the model to capture a broader range of linguistic patterns and nuances, potentially resulting in more accurate and contextually appropriate outputs.
Efficiency Through Technique:
Despite the larger training data size, Google states that PaLM 2 is a smaller model. This implies that Google has developed more efficient techniques to extract knowledge from the increased volume of data, reducing the model’s overall complexity while maintaining or even improving its performance. This focus on efficiency aligns with the industry’s pursuit of creating AI models that strike a balance between accuracy, computational resources, and environmental sustainability.
Transparency Concerns in AI Training Data:
While the expansion of training data in PaLM 2 appears promising, the lack of transparency surrounding the specifics of the data used raises concerns within the research community. Transparency in AI training data is crucial for various reasons, including bias detection and mitigation, accountability, and reproducibility of results. Researchers and experts argue that without transparency, it becomes challenging to identify potential biases or evaluate the model’s behavior accurately.
Addressing Transparency Challenges:
To address the growing demand for transparency, it is essential for organizations like Google to provide clearer insights into the data sources, collection methods, and pre-processing techniques used in training their AI models. Transparency initiatives, such as public datasets, data provenance documentation, and rigorous disclosure standards, can enhance trust in AI systems and facilitate more robust evaluations of their performance and fairness.
The Path Towards Responsible AI:
As AI models continue to advance and become increasingly integral to various aspects of society, the need for responsible AI development becomes paramount. Organizations must prioritize transparency, ethics, and inclusivity in their AI research and development processes. Collaboration between industry, academia, and regulatory bodies is crucial to establish guidelines and frameworks that promote transparency, fairness, and accountability in AI training.
Google’s PaLM 2 large language model represents a significant milestone in AI research.
It utilizing nearly five times more text data for training compared to its predecessor. While this expansion holds promise for improved language understanding and model performance, concerns surrounding transparency in AI training data persist. Addressing these concerns and promoting transparency initiatives are critical steps toward ensuring responsible AI development, fostering trust in AI systems, and facilitating informed evaluations of their impact. The ongoing dialogue between researchers, organizations, and regulators will shape the future of AI, paving the way for advancements that benefit society as a whole.
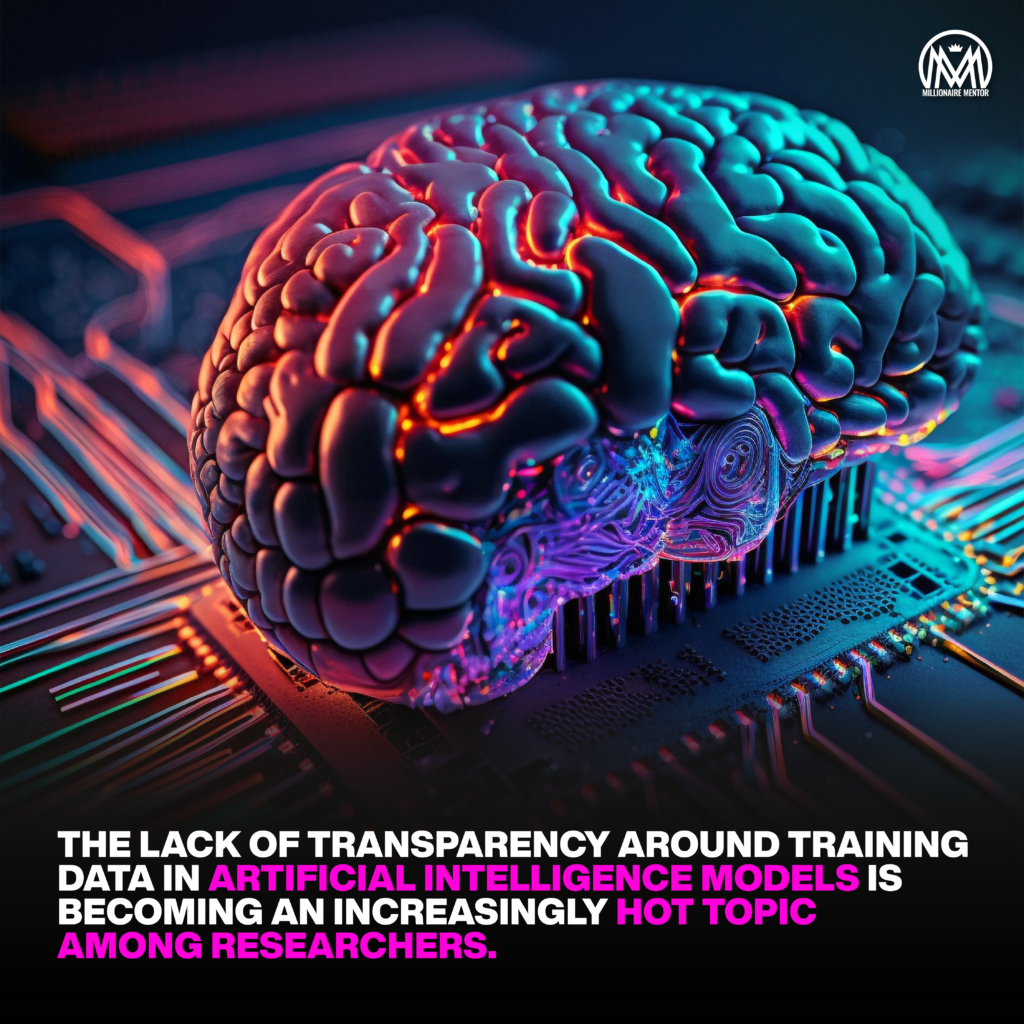
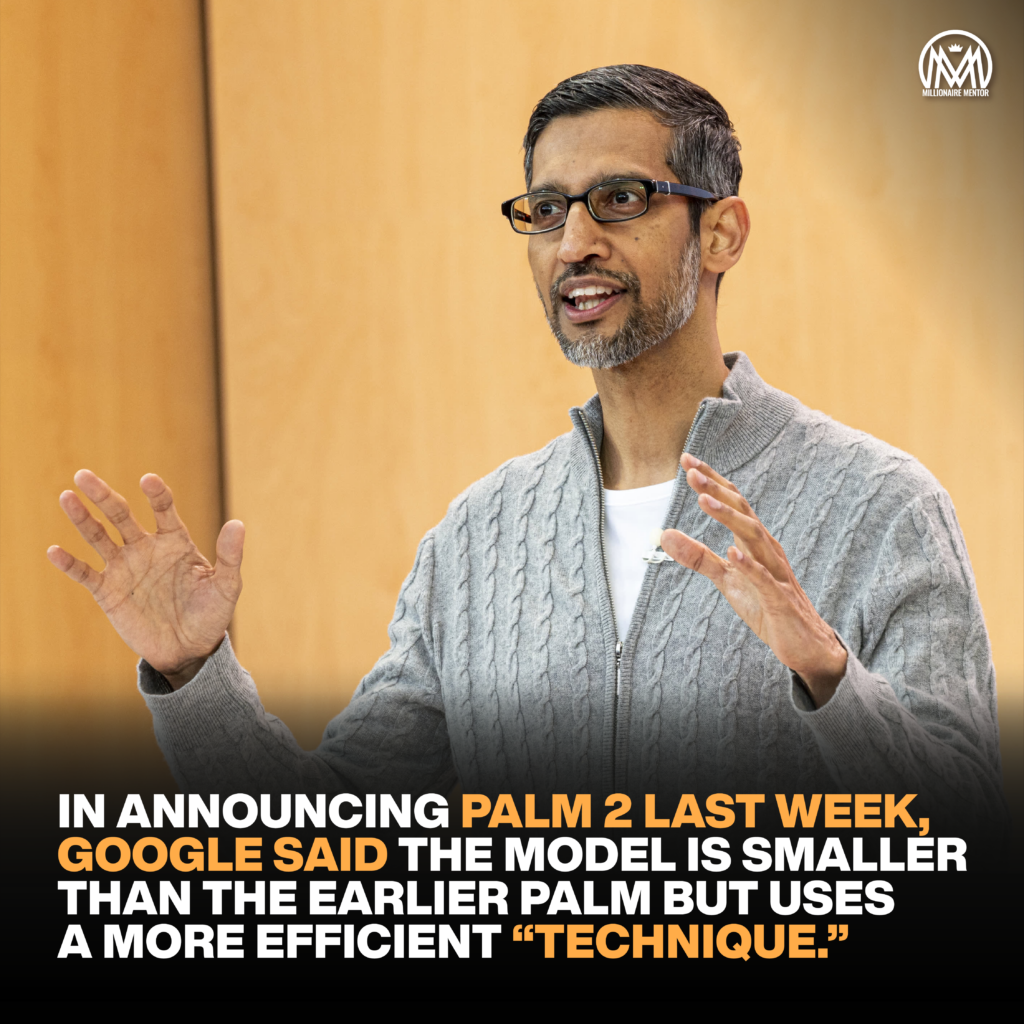
Trending News Articles
- The Chinese Dynasty Will Fall Before 2030 & Here’s Why | Peter Zeihanby Jason Stone●October 3, 2022
- Jordan Peterson: “The Survival Of 4 Billion People Depends On This”by Jason Stone●December 19, 2022
- AMD Hits US Roadblock in Selling AI Chip Tailored for Chinaby Jason Stone●March 5, 2024
- Future Proofing Your Success:by Jason Stone●March 6, 2017